Overview
The current research themes fall in the following categories:
- Data-Driven Computational Solid Mechanics for multiphase materials (e.g., engineered composites, biological tissues, geological materials), with a specific focus on modeling failure and other inelastic behaviors
- Multiscale and Multiphysics Simulation: Applications include chemo-electro-mechanical skeletal muscle modeling, renewable energy storage system, flow and transport in porous media
- Machine Learning Enhanced Computational Methods: Data assimilation, reduced-order modeling, and inverse problems
- AI for Science/Engineering: Develop scientific machine learning approaches to understand multiphysics processes across scales, ranging from composite and energetic materials to climate systems
- Hybrid Computing for Advanced Materials Design & Optimization
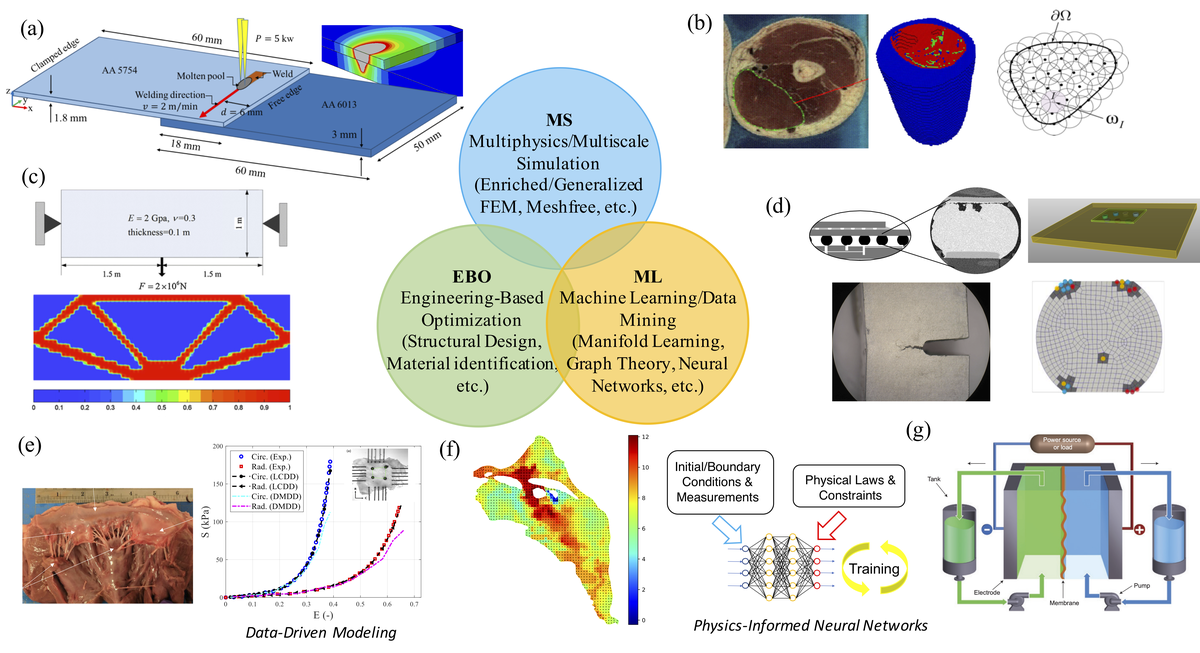
Research highlights. (a) MS: Thermomechanical analysis of lap-joint of Al alloys under laser welding process. (b) MS: Multiscale simulation of skeletal muscle tissues based on image pixel-based meshfree computational framework. (c) MS & EBO: Topological design of compliance structures (e.g. multi-physics actuators). (d) MS & ML: Reduced-order modeling for fatigue life prediction of viscoplastic solder joints in electronic packages based on hyper-reduced meshfree methods. (e) EBO & ML: Geomaterial properties estimation and hydraulic state prediction by physics-informed machine learning for the subsurface system of Hanford site (high-level nuclear waste-contaminated area). (f) MS & ML: Physics-constrained data-driven modeling of soft biological tissues.
Data-driven engineering and mechanics
Develop novel computational methods under an optimization framework that integrates scientific computing, data-driven machine learning, and particle-based methods to the modeling of soft tissues, fracture mechanics, and nonlinear solids.
References: He, X., He, Q. & Chen, J.S., Comput Methods Appl Mech Eng (2021); He, X., He, Q. & Chen, J.S., Data-Centric Engineering (2020); He, Q. et al., Journal of Biomechanics (2020); He, Q. & Chen, J.S., Comput Methods Appl Mech Eng (2019)
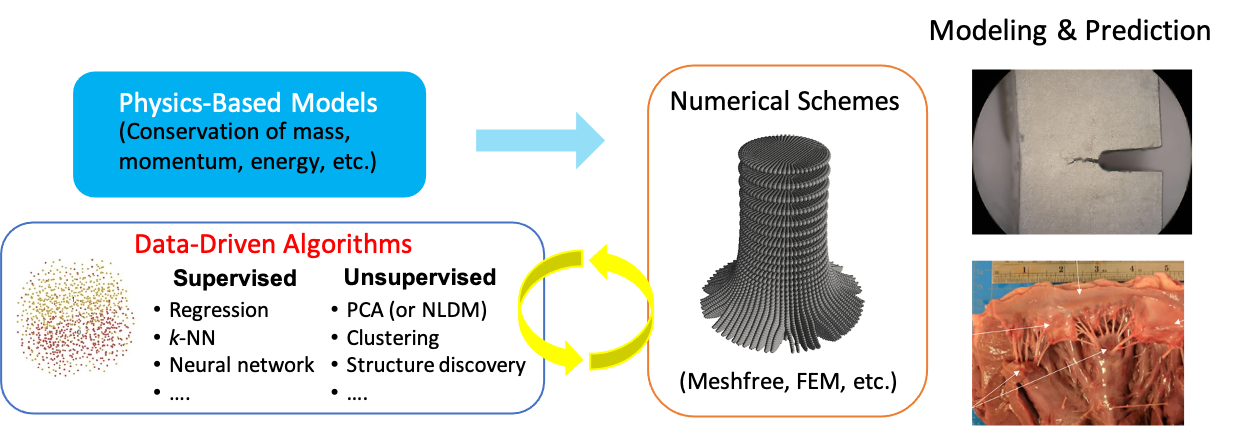
Physics-informed/Scientific machine learning
Develop effective and robust deep learning methods based on physics-informed neural networks to solve multiphysics problems arising in engineering science.
References: Du et al. Computers and Geotechnics (2023); He, Q. & Tartakovsky, A., Water Resources Research (2021); Tartakovsky, A., Barajas-Solano, D., He, Q., Journal of Computational Physics (2020); He, Q. et al. Advances in Water Resources (2020)
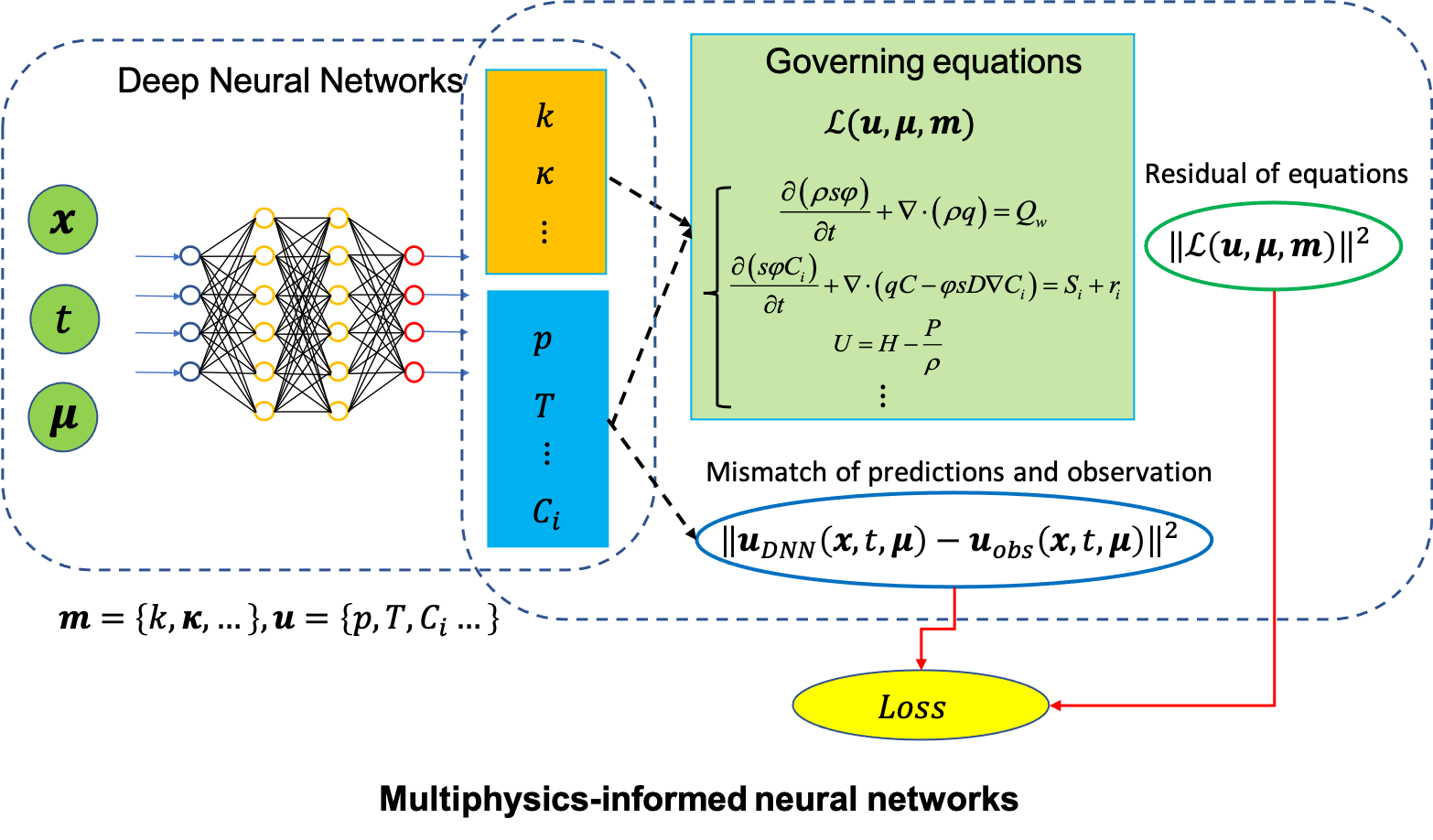
Modeling density-driven flow for CO2 sequestration
Ref: Du et al. (2023) Modeling density-driven flow in porous media by physics-informed neural networks for CO2 sequestration. Computers and Geotechnics.
FEM-SUPG
Enriched PINN with Domain Decompostion
Machine learning enhanced meshfree modeling, multiscale modeling, reduced-order modeling
Hyper reduced-order RKPM modeling for thermal fatigue in Electronic Devices
References: Kaneko, S., Wei, H., He, Q., et al., Journal of the Mechanics and Physics of Solids (2021); He, Q., Chen, J. S., & Marodon, C., Computational Mechanics (2019)
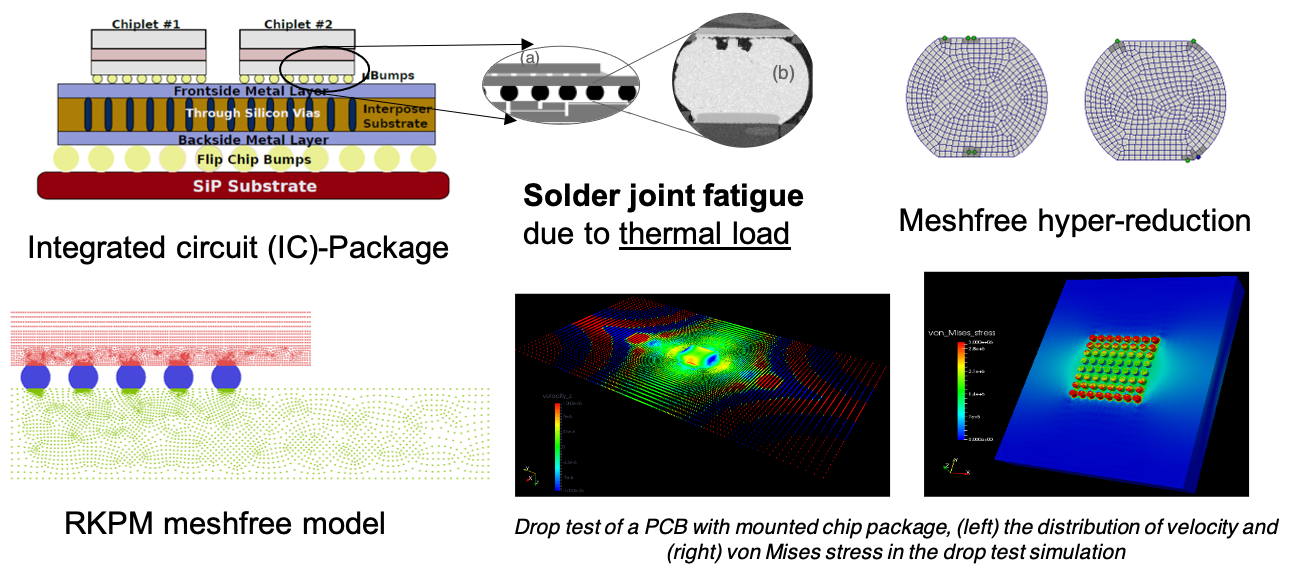
Geohazard modeling

Multiscale modeling of advanced materials and manufacturing
References: Zhang, Y. et al. Int J Numer Method Biomed Eng (2020); He, Q. et al. Int J Adv Manuf Technol (2018); Wei, H., He, Q. et al. Journal of Laser Applications (2017)
Deep learning and computational methods for material design
Reference: He, Q. et al. Computational Mechanics (2014); Wang Y. et al. Computers & Structures (2013, 2014)